Held in conjunction with Genomics Research Asia
15 Nov 2012 - 16 Nov 2012, at 09:00-17:00 in Daejeon, South Korea
The objective of this course is to provide an understanding of data processing and statistical methods applicable for analysis of data obtained by real-time PCR. This course is based on seminars and computer-based exercises.
Learning Objectives
After completed course students will know:
- How to estimate nucleic acid levels in test samples relative to standard samples and the precision of the estimate.
- How to determine the level of detection (LOD) of a qPCR based analytical procedure.
- How to compare the expression of targeted genes in two or more samples, and assess if the measured difference is statistically significant.
- How to identify the most confounding experimental steps, whose performance is most important to improve
- How to design qPCR experiment for optimum cost-performance
- How to estimate the number of subjects needed in a study in order to be able to detect a certain treatment effect against the background of confounding variation.
- How to select optimum normalization strategy.
- How to identify patterns among expressed genes in profiling studies
- How to separate sample classes in multimarker expression profiling studies
- The principles of multimarker molecular diagnostics
Topics and Course Organization
- Introduction to qPCR theory, ?Cq and ??Cq
- Absolute quantification, qPCR standard curve, Reverse calibration, Limit of detection
- Experimental design, Noise contributions to RT-qPCR analysis (nested ANOVA), cost-performance optimization of experiments
- Sample size estimations (Power testing)
- Selecting reference genes (geNorm, Normfinder)
- Relative quantification, qPCR data pre-processing, Outlier detection. Comparison of groups (parametric and non-parametric methods)
- Expression profiling, missing data treatment, scaling of data, Un-supervised clustering of genes and samples (hierarchical clustering, self-organized maps, Principal Component Analysis), Supervised clustering of samples
- Exercises
|
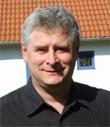 Mikael Kubista, Professor/Founder, TATAA Biocenter AB
|